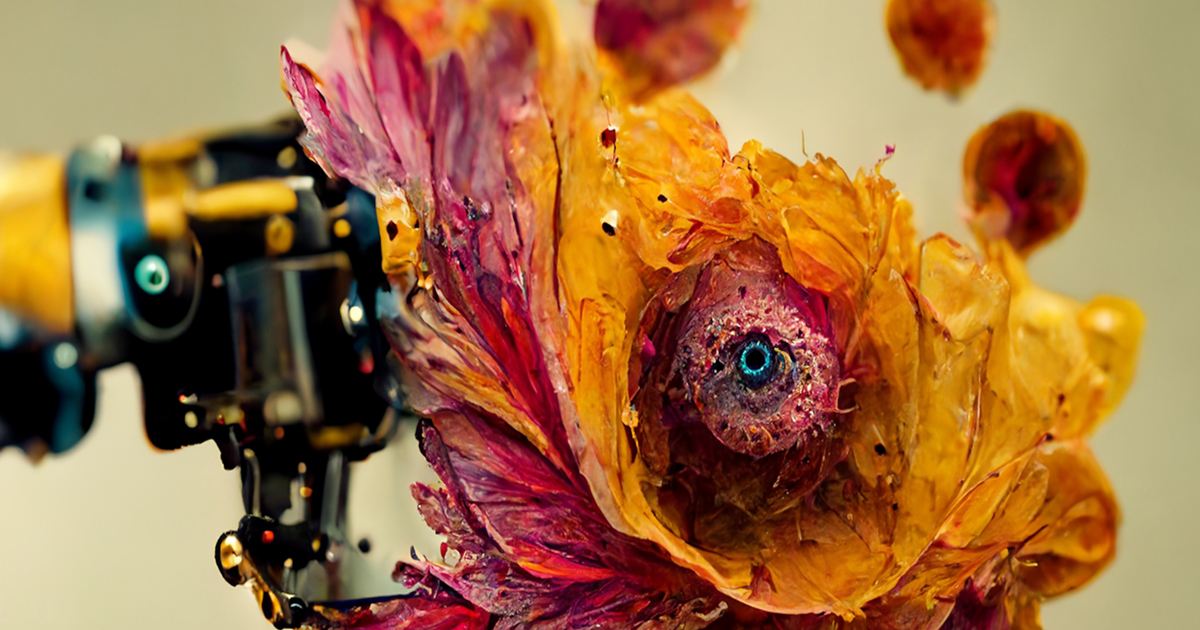
TL;DR
- Diffusion models replaced GANs (generative adversarial networks) to drive the recent trend in generative AI tools.
- Diffusion-based AI has also proved adept at composing music and video.
- The tech has been around for a decade but it wasn’t until OpenAI developed CLIP (Contrastive Language-Image Pre-Training) that diffusion became practical in everyday applications.
READ MORE: A brief history of diffusion, the tech at the heart of modern image-generating AI (TechCrunch)
Text-to-image AI exploded last year as technical advances greatly enhanced the fidelity of art that AI systems could create. At the heart of these systems is a technology called diffusion, which is already being used to auto-generate music and video.
So what is diffusion, exactly, and why is it such a massive leap over the previous state of the art? Kyle Wiggers has done the research at TechCrunch.
We learn that earlier forms of AI technology relied on generative adversarial networks, or GANs. These proved pretty good at creating the first deepfaking apps. For example, StyleGAN, an NVIDIA-developed system, can generate high-resolution head shots of fictional people by learning attributes like facial pose, freckles and hair.
READ MORE: Can you guess which face is real, and which is computer generated? (TechCrunch)
In practice, though, GANs suffered from a number of shortcomings owing to their architecture, says Wiggers. The models were inherently unstable and also needed lots of data and compute power to run and train, which made them tough to scale.
Diffusion rode to the rescue. The tech has actually been around for a decade but it wasn’t until OpenAI developed CLIP (Contrastive Language-Image Pre-Training) that diffusion became practical in everyday applications.
CLIP classifies data — for example, images — to “score” each step of the diffusion process based on how likely it is to be classified under a given text prompt (e.g. “a sketch of a dog in a flowery lawn”).
Wiggers explains that, at the start, the data has a very low CLIP-given score, because it’s mostly noise. But as the diffusion system reconstructs data from the noise, it slowly comes closer to matching the prompt.
“A useful analogy is uncarved marble — like a master sculptor telling a novice where to carve, CLIP guides the diffusion system toward an image that gives a higher score.”
OpenAI introduced CLIP alongside the image-generating system DALL-E. Since then, it’s made its way into DALL-E’s successor, DALL-E 2, as well as open source alternatives like Stable Diffusion.
So what can CLIP-guided diffusion models do? They’re quite good at generating art — from photorealistic imagery to sketches, drawings and paintings in the style of practically any artist.
Researchers have also experimented with using guided diffusion models to compose new music. Harmonai, an organization with financial backing from Stability AI, the London-based startup behind Stable Diffusion, released a diffusion-based model that can output clips of music by training on hundreds of hours of existing songs. More recently, developers Seth Forsgren and Hayk Martiros created a hobby project dubbed Riffusion that uses a diffusion model cleverly trained on spectrograms — visual representations — of audio to generate tunes.
READ MORE: Try ‘Riffusion,’ an AI model that composes music by visualizing it (TechCrunch)
Researchers have also applied it to generating videos, compressing images and synthesizing speech. Diffusion may be replaced with a more efficient machine learning technique but the exploration has only just begun.
READ MORE: Video Diffusion Models (arXivLabs)
READ MORE: Better than JPEG? Researcher discovers that Stable Diffusion can compress images (Ars Technica)
READ MORE: FastDiff: A Fast Conditional Diffusion Model for High-Quality Speech Synthesis (arXivLabs)
Next, Watch This
AI ART — I DON’T KNOW WHAT IT IS BUT I KNOW WHEN I LIKE IT:
Even with AI-powered text-to-image tools like DALL-E 2, Midjourney and Craiyon still in their relative infancy, artificial intelligence and machine learning is already transforming the definition of art — including cinema — in ways no one could have ever predicted. Gain insights into AI’s potential impact on Media & Entertainment in NAB Amplify’s ongoing series of articles examining the latest trends and developments in AI art
- What Will DALL-E Mean for the Future of Creativity?
- Recognizing Ourselves in AI-Generated Art
- Are AI Art Models for Creativity or Commerce?
- In an AI-Generated World, How Do We Determine the Value of Art?
- Watch This: “The Crow” Beautifully Employs Text-to-Video Generation