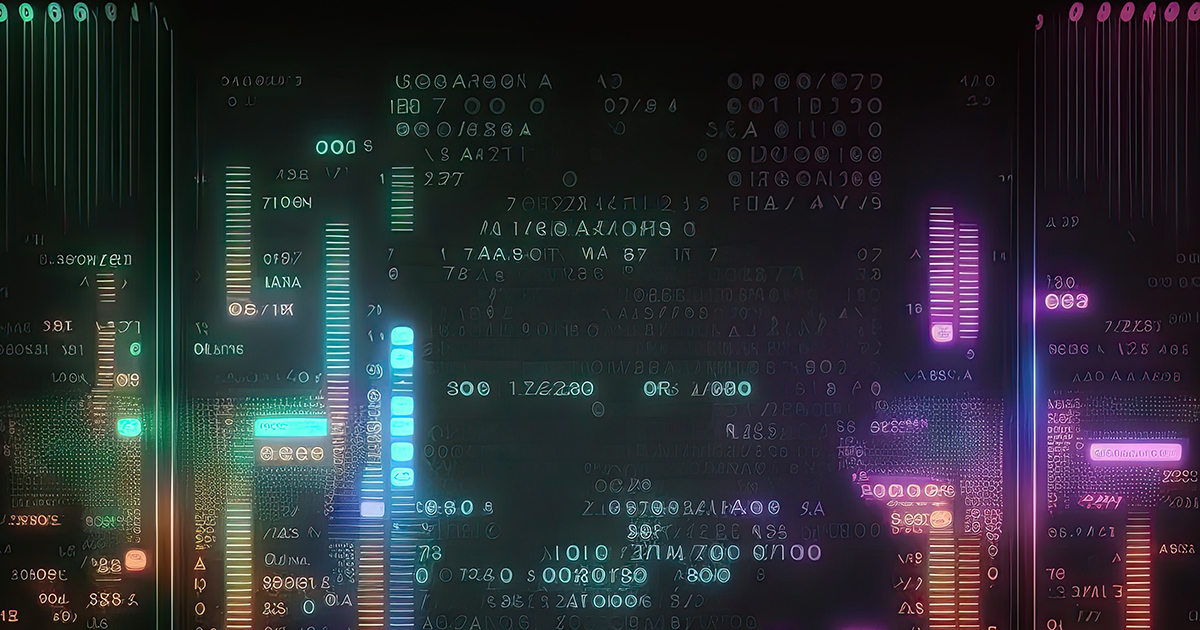
TL;DR
- The most promising future directions and research trends in Large Language Models (LLMs) offers a glimpse into the exciting developments that lie ahead.
- These include the integration of LLMs with other AI disciplines, such as computer vision to facilitate tasks like visual storytelling, image captioning, and human-robot interaction.
- The future of LLM research will likely emphasize the development of more interpretable and transparent models, enabling users to better understand and trust AI-driven decisions.
READ MORE: Unveiling the Power of Large Language Models (LLMs) (Unite.AI)
The development of Large Language Models (LLMs) has already significantly impacted the field of natural language processing and artificial intelligence but research has barely begun.
Already, work is underway to create even larger and more powerful LLMs. These efforts aim to tackle the challenges associated with scaling, such as memory limitations, to enable the development of next-generation LLMs.
What might they look like? Here are five strong clues, courtesy of futurist Antoine Tardif, writing at Unite.AI.
Multimodal Learning and Integration
Future LLM research is expected to focus on multimodal learning, where models are trained to process and understand multiple types of data, such as text, images, audio, and video. By incorporating diverse data modalities, LLMs can gain a more holistic understanding of the world and enable a wider range of AI applications.
The convergence of LLMs with other AI disciplines, such as computer vision and reinforcement learning, is expected to follow. These integrated models can facilitate tasks like visual storytelling, image captioning, and human-robot interaction, unlocking new possibilities in AI research and applications.
Personalization and Adaptability
Researchers are exploring ways to adapt LLMs to individual users’ needs, preferences, and contexts, creating more personalized and effective AI-driven solutions. Techniques like fine-tuning, meta-learning, and federated learning can be employed to tailor LLMs to specific users, tasks, or domains, offering a more customized and engaging user experience.
Another area of interest is the development of LLMs capable of continual and lifelong learning, enabling them to adapt and evolve over time as they interact with new data and experiences. This adaptability can help LLMs remain relevant and effective in dynamic and ever-changing environments.
Ethical AI and Trustworthy LLMs
As the ethical implications of LLMs gain increasing attention, researchers are focusing on developing techniques to identify, quantify, and mitigate biases in these AI systems. The goal is to create more equitable and fair LLMs that do not perpetuate harmful stereotypes or discriminatory outcomes.
The future of LLM research will likely emphasize the development of more interpretable and transparent models, enabling users to better understand and trust AI-driven decisions. Techniques like attention visualization, feature attribution, and surrogate models can be employed to enhance the explainability of LLMs and foster trust in their outputs.
Cross-Lingual Modelling
The development of LLMs capable of understanding and generating text in multiple languages is a promising research direction. Cross-lingual learning can enhance the accessibility and usefulness of LLMs, bridging language barriers and enabling more inclusive AI applications that cater to diverse linguistic communities.
Robustness and Adversarial Defense
Ensuring the robustness of LLMs against adversarial attacks, data distribution shifts, and other potential sources of uncertainty is an essential aspect of future research. Developing techniques to improve model robustness and resilience will contribute to the deployment of more reliable and trustworthy AI solutions.
Researchers are exploring methods to defend LLMs against adversarial attacks, such as adversarial training, input sanitization, and model verification. These efforts aim to enhance the security and stability of LLMs, ensuring their safe and dependable operation in real-world applications.
Tardif concludes that the future of LLMs promises exciting advancements and research breakthroughs that will further expand the capabilities and applications of AI systems.
“By focusing on areas such as model efficiency, multimodal learning, personalization, ethical AI, and robustness,” he says, “the AI research community will continue to push the boundaries of what LLMs can achieve, paving the way for a new era of AI-driven innovation that benefits users and society at large.”