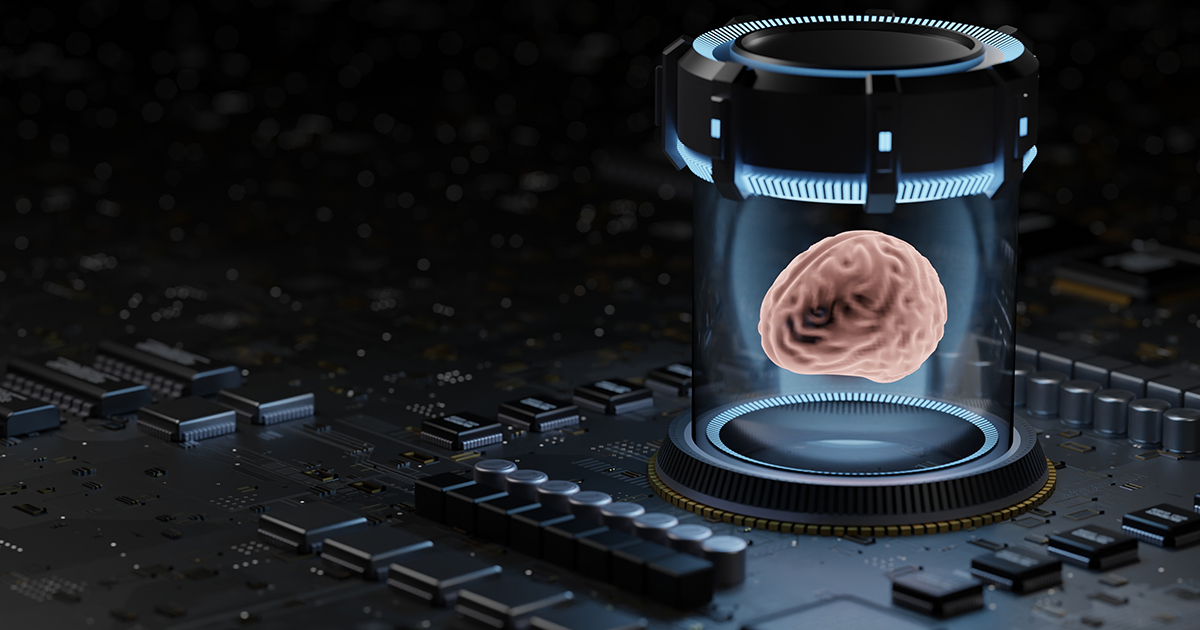
READ MORE: What the history of AI tells us about its future (MIT Technology Review)
The science of artificial intelligence is new but has already taken a few twists and turns. There’s a debate raging in some quarters that computers alone will never have the smarts to emulate human thought — unless we work in collaboration with the machine.
The idea requires a brief history of AI, which Clive Thompson charts in the MIT Technology Review. Go back to 1997, when IBM computer Deep Blue made headlines by beating chess grandmaster Gary Kasparov. Game over — or so everyone thought. In fact, not long afterwards, Deep Blue was left out in the cold.
“Deep Blue’s victory was the moment that showed just how limited hand-coded systems could be. IBM had spent years and millions of dollars developing a computer to play chess. But it couldn’t do anything else,” says Thompson.
The reason lay the in AI baked into Deep Blue. It could play chess, brilliantly, because it’s based on logic: the rules are clear, there’s no hidden information, and a computer doesn’t even need to keep track of what happened in previous moves. It just assesses the position of the pieces right now.
Chess turned out to be fairly easy for computers to master. What was far harder for computers to learn was the casual, unconscious mental work that humans do — ”like conducting a lively conversation, piloting a car through traffic, or reading the emotional state of a friend.”
This requires, in Thompson’s phraseology “fuzzy, grayscale judgment,” which we do without thinking.
Enter the era of neural nets.
Instead of hard-wiring the rules for each decision, a neural net trained and reinforced on data would strengthen internal connections in rough emulation of how the human brain learns.
By the 2000s, the computer industry was evolving to make neural nets viable and, by 2010, AI scientists could create networks with many layers of neurons (which is what the “deep” in “deep learning” means).
“The future lies in finding ways to combine human and machine intelligences to reach new heights, and to do things neither could do alone. We will increasingly become managers of algorithms and use them to boost our creative output — our adventuresome souls.”
Gary Kasparov
A decade into our deep-learning revolution and neural nets and their pattern-recognizing abilities have colonized every nook of daily life.
Writes Thompson, “They help Gmail autocomplete your sentences, help banks detect fraud, let photo apps automatically recognize faces, and — in the case of OpenAI’s GPT-3 and DeepMind’s Gopher — write long, human-sounding essays and summarize texts.”
“Deep learning’s great utility has come from being able to capture small bits of subtle, unheralded human intelligence,” he says.
Yet Deep Learning’s position as the dominant AI paradigm is coming under attack. That’s because such systems are often trained on biased data.
For instance, computer scientists Joy Buolamwini and Timnit Gebru discovered that three commercially available visual AI systems were terrible at analyzing the faces of darker-skinned women.
On top of that, neural nets are also “massive black boxes,” according to Daniela Rus, who runs MIT’s Computer Science and AI Lab. Once a neural net is trained, its mechanics are not easily understood even by its creator, she says. It is not clear how it comes to its conclusions — or how it will fail.
This manifests itself in real world problems. For example, visual AI (computer vision) can make terrible mistakes when it encounters an “edge” case.
“Self-driving cars have slammed into fire trucks parked on highways, because in all the millions of hours of video they’d been trained on, they’d never encountered that situation,” according to Thompson.
Some computer scientists believe neural nets have a design fault and that the AI also needs to be trained in common sense.
In other words, a self-driving car cannot rely only on pattern matching. It also has to have common sense — to know what a fire truck is, and why seeing one parked on a highway would signify danger.
The problem is that no one quite knows how to build neural nets that can reason or use common sense.
Gary Marcus, a cognitive scientist and co-author of Rebooting AI, tells Thompson that the future of AI will require a “hybrid” approach — neural nets to learn patterns, but guided by some old-fashioned, hand-coded logic. This would, in a sense, merge the benefits of Deep Blue with the benefits of deep learning.
Then again, hardcore aficionados of deep learning disagree. Scientists like Geoff Hinton, an emeritus computer science professor at the University of Toronto, believes neural networks should be perfectly capable of reasoning and will eventually develop to accurately mimic how the human brain works.
Still others argue for a Frankensteinian approach — the two stitched together.
One of them is Kasparov, who after losing to Deep Blue, invented “advanced chess,” where humans compete against each other in partnership with AIs.
Amateur chess players working with AIs (on a laptop) have beaten superior human chess pros. This, Kasparov argues in an email to Thompson, is precisely how we ought to approach AI developments.
“The future lies in finding ways to combine human and machine intelligences to reach new heights, and to do things neither could do alone,” Kasparov says. “We will increasingly become managers of algorithms and use them to boost our creative output — our adventuresome souls.”